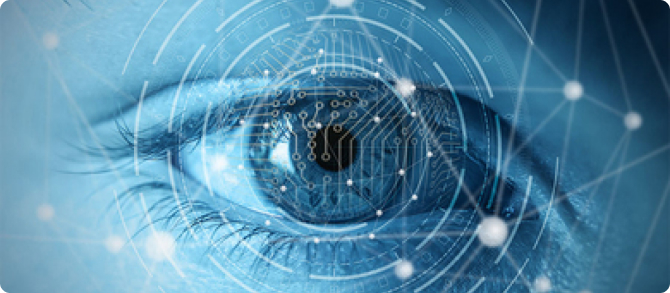
From cameras to self-driving cars, many of today’s technologies depend on artificial intelligence to extract meaning from visual information. Today’s AI technology has artificial neural networks at its core, and most of the time we can trust these AI computer vision systems to see things the way we do — but sometimes they falter.
According to MIT and IBM research scientists, one way to improve computer vision is to instruct the artificial neural networks that they rely on to deliberately mimic the way the brain’s biological neural network processes visual images.
Researchers led by MIT Professor James DiCarlo, the director of MIT’s Quest for Intelligence and member of the MIT-IBM Watson AI Lab, have made a computer vision model more robust by training it to work like a part of the brain that humans and other primates rely on for object recognition. This May, at the International Conference on Learning Representations, the team reported that when they trained an artificial neural network using neural activity patterns in the brain’s inferior temporal (IT) cortex, the artificial neural network was more robustly able to identify objects in images than a model that lacked that neural training.
And the model’s interpretations of images more closely matched what humans saw, even when images included minor distortions that made the task more difficult.
Comparing neural circuits
Many of the artificial neural networks used for computer vision already resemble the multilayered brain circuits that process visual information in humans and other primates. Like the brain, they use neuron-like units that work together to process information. As they are trained for a particular task, these layered components collectively and progressively process the visual information to complete the task — determining, for example, that an image depicts a bear or a car or a tree.
DiCarlo and others previously found that when such deep-learning computer vision systems establish efficient ways to solve visual problems, they end up with artificial circuits that work similarly to the neural circuits that process visual information in our own brains.
That resemblance is helping neuroscientists deepen their understanding of the brain. By demonstrating ways visual information can be processed to make sense of images, computational models suggest hypotheses about how the brain might accomplish the same task.
“As vision systems get better at performing in the real world, some of them turn out to be more human-like in their internal processing. That’s useful from an understanding-biology point of view,” says DiCarlo, who is also a professor of brain and cognitive sciences and an investigator at the McGovern Institute for Brain Research.